How AI Impacts Quality of Hire.
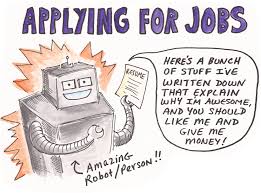
Quality of hire is arguably the most valuable, yet least collected - and least understood, of the manifold metrics leveraged in recruitment and hiring today.
Now, I say it's the most valuable metric because, simply, it provides the most clear and concise answer to the most important question - and fundamental purpose - of recruiting:
Am I actually hiring the right people?
You'd think that every employer out there would demand some sort of empirical evidence or quantitative baseline so that they can accurately answer this seemingly simple and straightforward question.
Yet, a recent LinkedIn report suggests only about 1 in 3 companies collect any sort of data related to measuring or managing quality of hire.
This is a fairly surprising statistic, considering the critical importance of quality of hire in recruitment and talent acquisition.
On the other hand, there are a ton of barriers to accurately measuring quality of hire.
First, measuring quality of hire requires some sort of statistical knowledge, which is rarely a competency we've historically hired for in the recruiting function.
Second, this talent acquisition specific skills gap is compounded by the many restrictions or challenges that exist in accessing and analyzing both pre and post-hire data.
Third, accurately measuring quality of hire data requires making a long term investment, since generating meaningful metrics, much less actionable analytics, can take literally years - anathema in a function where recruiters are mainly measured by how many days it takes to fill an open requisition.
These are all contributing factors to why quality of hire data is so rarely measured, even though most talent leaders are aware of its critical importance in analyzing hiring success (or lack thereof) in any meaningful way.
However, with the competition for top talent becoming increasingly cutthroat due to record employment and a growing skills gap, there's been more pressure than ever before for recruiting departments to quantify their strategic value.
Increasingly, recruiters are being required to provide data to demonstrate not only their relative ROI, but also, how those efforts impact the bigger business picture and the company's bottom line.
It's this imperative to prove strategic value, coupled with the dearth of data or analytic acumen required to do so, that suggest the demand to prove quality of hire will only continue to grow, at least for the foreseeable future.
How To (Really) Measure Quality of Hire
Today, however, most companies haven't even taken the first step required to calculate quality of hire, which is to categorically define what "quality" really means to your company. While the concept of "quality" is often subjective at many employers, standardization is crucial for measuring, monitoring or managing any metric related to quality of hire.
with the business as a first step in developing quality of hire data is also critical because this approach requires both sides to compromise on leveraging metrics that both recruiters and hiring managers actually trust. For example, in some cases, employers will dismiss formal employee reviews or supervisor ratings as inflated, and more driven by internal politics than actual job performance.
For some roles, of course, objective performance data is relatively straightforward, statistically speaking. For example, sales has long been measured by KPIs such as total annual sales, commissions earned, YoY growth and the number of new clients acquired or retained in any given period. Similarly, call center workers can be measured by their call volume, resolution time and customer satisfaction scores or feedback.
For most roles, however, hard data doesn't yet exist, although there is unilaterally a threshold where individual employees start adding value. In addition to common quality of hire metrics such as an employee's annual performance, job tenure or individual productivity, the ability to calculate or estimate the financial impact is a major step for recruiters to demonstrate how they've directly contributed to the company's bottom line or bigger business objectives.
Quantifying the value a hire adds to an organization creates a baseline for calculating their financial impact on your organization.
The formula is pretty simple, actually:
Financial impact = Value created by the hire / Recruiting & employment costs
With this basic equation in mind, you should be able to accurately calculate quality of hire and associated metrics, but of course, this raises another significant issue. Where, exactly, does automation or AI fit into the equation?
While AI and intelligent automation have obvious applications for recruiter efficiency, AI also holds the intriguing potential to make a big impact on quality of hire.
Quality of Hire and AI: The Perfect Match.
Probably the most significant potential promised by AI for improving quality of hire lies in the concept of machine learning; that is, the ability of these algorithms to use data to learn and improve its matching capabilities between job requirements and candidates' qualifications.
At the front end of the hiring process, machine learning software can intrinsically understand the requisite skills, experience and job qualifications currently exist within your workforce, and leverages this historical data to automatically grade and display those external candidates whose profiles most closely match internal requirements.
Finding the best fit isn't an art; it's data science.
Of course, the potential impact of intelligent automation on how companies hire extends far past the initial prescreening process. There are, in fact, applications for machine learning enabled technologies throughout the entire talent life cycle.
From sourcing to screening, from selection to succession, AI can be effectively applied to integrate the many disparate sources and systems of talent analytics into a simple, streamlined solution.
In recruiting, specifically, AI can be used to combine historical data from resumes, assessments, interviews with new hire and/or performance management data. This allows recruiters to know how each candidate stacks up relative to every other record currently housed in your system of record, whether that's an ATS, an HCM or ERP solution.
Machine learning can essentially predict whether or not a candidate will even make it through the hiring process, and how successful they're likely to be in the role before a recruiter even looks at their resume.
Translation: recruiters, you'll never have to waste time on phone screens or reviewing resumes ever again. And, of course, all this automation means that you can focus your efforts on personalization, instead.
Because in the business of human capital, the human touch still can't be replaced by high tech.
But it sure can help.
To learn more about how AI can make a tangible impact on your recruitment performance, click here.